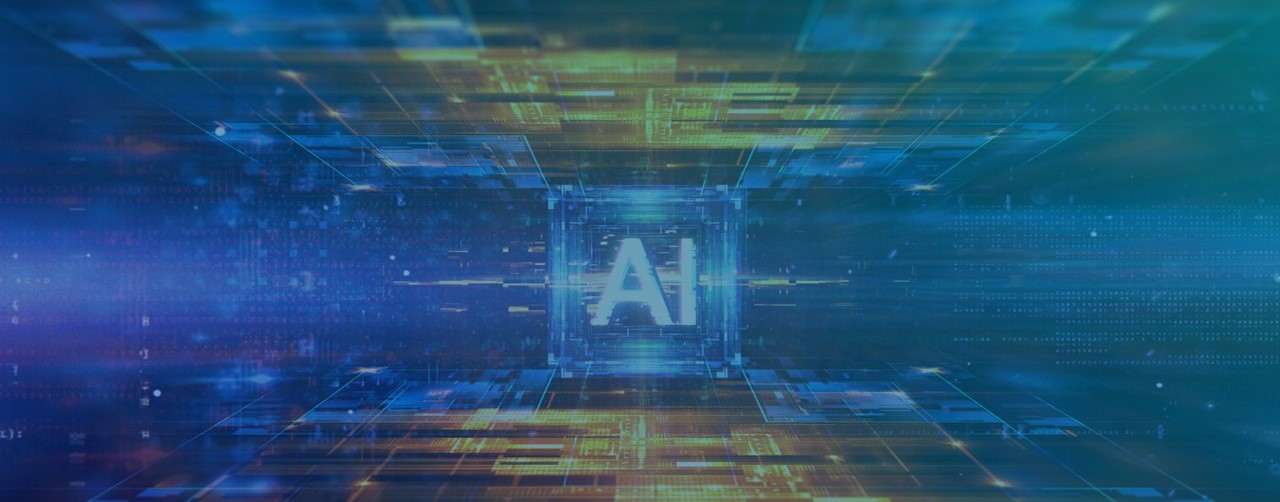
While the existence and usage of various forms of artificial intelligence (AI) is not new, in recent years the adoption of AI has accelerated, while the modes of deployment have evolved. These changes are leading to new dynamics that increase the risks of cyber event aggregation, arising from both malicious sources and accidental ones.
Artificial Intelligence: A multi-pronged driver of cyber aggregation risk
In this paper, we discuss 4 primary ways AI development and deployment lead to this aggregation: 1) software supply chain risk, 2) expansion of the attack surface, 3) increased data exposure and 4) growing usage in cyber security operations. While this presents new challenges, it also creates new opportunities for the entire insurance ecosystem—from carriers to reinsurers—to learn, design and optimize risk management solutions for their respective stakeholders.
AI presents an additional software supply chain threat
For businesses to use AI, it must be deployed. This can happen in a variety of ways. For firms using third-party solutions, such as ChatGPT, Claude or others, AI models can be deployed within the customer’s network (a walled- off solution) or hosted externally and used by sending requests to the third-party provider model itself.
In both cases, a compromise or degradation of the third- party model presents a risk to downstream customers. The AI vendor in this case becomes a single point of failure for all customers using their AI models. This failure can manifest as an outage, a compromise or both. In the short time that large AI models have been available, there have been multiple instances of availability or integrity compromises:
- In 2023 and 2024, ChatGPT suffered several outages, affecting thousands of users. [1]
- On December 25, 2022, PyTorch, a popular machine learning library, was compromised via a software supply chain attack, leading to at least 2,300 malicious downloads. [2]
- More than 100 malicious AI models were discovered on HuggingFace, a popular AI repository. [3]
AI presents a new attack surface
Once deployed, users can interact with a model. Whether it is a chatbot, a mortgage calculator or a customized image analysis model, the model receives inputs and sends outputs. This process is subject to malicious and sometimes accidental manipulation.
“Jailbreaking” is a common term for ways to “trick” a model into behaving outside its intended boundaries. Like conventional software exploitation, this can lead to data exposure, loss of availability or even exploitation leading to a network breach. In addition to this new attack vector, AI models may simply provide wrong answers—which can lead to liability. This highlights a unique threat to AI compared to conventional software: even when the code is functional and secure, if the outputs are wrong and there is no check in place, it can produce failures that can lead to substantial consequences for a firm. For example:
- In February 2024, attackers used a vulnerability in an open-source library to steal information from other users’ ChatGPT interactions. [4]
- Air Canada paid damages to a passenger after the airline’s AI chatbot gave incorrect information to the passenger. [5]
- Zillow wrote off USD 304 million in inventory from home- buying decisions made by the Zillow Offers algorithm. [6]
AI presents a data privacy threat
It is often said that a model is only as good as the data on which it is trained. In order to train your own models, or to fine-tune third-party models, those models must be given access to relevant datasets—often large, sensitive datasets. Thus, data must be exposed, replicated or otherwise made available to these training pipelines. Indeed, this has led to an entire economy of data storage and engineering vendors, and these solutions are not without risk.
In many cases, the success of AI technology is tied directly to the scale of the data to which it has access. The more customers, the more datasets, the more deployments a given AI vendor has, the better its solution tends to become. This creates a strong incentive for aggregation— both for the AI vendor and for their customers. The quality of the product improves as the customer population grows. AI development favors centralization and aggregation, and with it, brings increased aggregation risk. Compromise to centralized storage, computation or training solutions can have dramatic downstream effects. For instance:
- In September 2023, AI researchers accidentally exposed 38 terabytes of customer data through a misconfiguration. [7]
- Beginning in April 2024, Snowflake, a data storage and processing vendor, experienced a breach affecting nearly 200+ customers and millions of users. [8]
AI in security roles
One of the highly touted use cases for AI is in cyber security operations—the very type of procedures that require high-level privileges, such as those present in CrowdStrike’s recent faulty software update. While there is no evidence that suggests AI played a role in that event, the expected usage of AI in the security supply chain presents similar risks. As development pipelines seek efficiencies in automation, the potential for errors, misconfigurations and vulnerabilities may increase.
Take, for example, the implementation of AI in security response orchestration. As ransomware attackers look for ways to increase leverage on victims, there is an imperative to remediate intrusions before impact has increased. Security products now have the capability to quarantine systems, cut off network access and reset credentials without a human in the loop. This “machine speed” response is an important tool for security practitioners, but it requires careful consideration given the dramatic effects these administrative actions can have. Indeed, a recent outage at Microsoft due to a malicious DDoS attack may have been exacerbated by Microsoft’s own response [9]. We must be careful not to make the medicine worse than the disease.
Empowering AI with response decisions may be a tempting straw to grasp in desperation, but if improperly managed, it introduces considerable risk. Guardrails, checks and bypasses must be made available to human responders to allow for shutdown or reversal of AI-based security decisions. These measures must also be implemented without compromising the security of the systems themselves.
Responsible vendors will heed this danger, but in a market that has historically rewarded growth and features over stability, some may not. To be sure, AI is a powerful technology; it would be foolish not to use it to fight malicious activity or predict and respond to outages and errors. However, it would be equally foolish to trust AI in critical networks without boundaries.
Looking ahead
As companies across the economic spectrum embrace AI technology in their product innovation and business operations, AI’s transformative effect on the way we work means it will inevitably change the future (re)insurance landscape as well. (Re)insurers should see this as an opportunity for growth rather than a risk to avoid.
The move to generate and deploy AI solutions has some similarities to the movement to cloud services a decade ago. Like the transition to cloud, AI adoption presents tremendous opportunities, but also introduces reliance on third parties.
In addition to relying on cloud infrastructure for storage, hosting and computation, companies are now asking third parties to manage data processing, decisions and dialogue. Vendors in the data engineering, machine learning and AI model markets, meanwhile, will seek to aggregate as much data as they can to improve their solutions for all customers. However, this increased risk is neither intractable nor immeasurable. It can and should be evaluated by examining AI deployment through a lens of third-party risk.
As the first step of underwriting and managing AI portfolio risk, (re)insurers should ask detailed questions and collect robust data about their insureds’ AI model development, deployment and testing:
- What checks exist to ensure confidentiality, integrity and availability?
- Are sensitive datasets aggregated for research and, if so, how is that data secured?
- Are AI solutions part of the security infrastructure and, if so, how are privileges managed and monitored?
We have already seen how the answers to these questions can mean the difference between compromise and confidence. Leveraging this data allows (re)insurers to better understand this risk, underwrite AI exposures profitably and manage aggregation risk as AI-related insurance volume scales with technological advancements.
Artificial Intelligence: A multi-pronged driver of cyber aggregation risk
Authors:
Matthew Berninger, Senior Vice President and Principal Cyber Analyst, Marsh McLennan Cyber Risk Intelligence Center
MJ Teo, Vice President and Senior Cyber Actuary, Guy Carpenter
Jess Fung, Managing Director and North American Cyber Analytics Lead, Guy Carpenter
Sources:
1. https://www.theverge.com/2024/6/4/24171264/chatgpt-down-outage-errors
4. https://securityintelligence.com/articles/chatgpt-confirms-data-breach/
5. https://www.theregister.com/2024/02/15/air_canada_chatbot_fine/
6. https://www.cio.com/article/190888/5-famous-analytics-and-ai-disasters.html